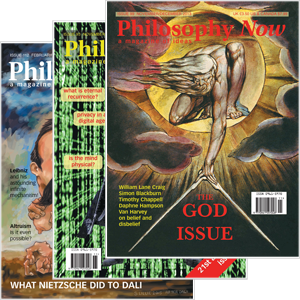
Your complimentary articles
You’ve read one of your four complimentary articles for this month.
You can read four articles free per month. To have complete access to the thousands of philosophy articles on this site, please
AI & Mind
What’s Stopping Us Achieving Artificial General Intelligence?
A. Efimov, D. Dubrovsky, and F. Matveev explore how the development of AI is limited by the perceived need to understand language and be embodied.
Over seventy years ago, Alan Turing developed the simple but powerful idea that any solvable mathematical problem can in principle be solved with a ‘universal computing device’. The type of device he described in his 1936 paper became known to researchers as a ‘Turing machine’. Ever since, we have been trying to create artificial intelligence by programming electronic machines. Most of the current research in the field of AI is indeed just an acceleration of that first universal Turing machine. Turing is also responsible for another fundamental idea that has shaped research in this area. The Turing test makes us ask: if we cannot distinguish whether we are holding a dialogue with a person or a machine, then does it really matter what is in front of us – a machine or a human – since we’re dealing with intelligence anyway?
The Merriam-Webster Dictionary defines intelligence as ‘the ability to learn or understand or to deal with new or trying situations’. Turing’s idea of using language as a tool for comparing machine and human intelligence, based on how well a machine can pretend to be human, is both simple and profound. Thanks to this idea, such wonderful things as voice assistants and online translators have come to life.
Modern developments are now getting close to the point when a single computer can tackle any problem, thus resembling a human being in the broadness of the application of its intelligence. This is called artificial general intelligence (AGI), which is also sometimes called ‘strong AI’. The idea is, the better and more accurate the means we employ to improve a program, the better it ‘understands’ our words, and the closer we approach artificial general intelligence. But what if this basic assumption is wrong? What if it is not just language that determines the ‘generality’ or the ‘intelligence’ of an artificial agent? Is there a possibility that the signpost planted by Turing (and not only by him) seventy years ago is pointing in the wrong direction, and we should reconsider our route? In this article we want to put forward a number of ideas in the philosophy of artificial intelligence. These ideas could offer conceptual support for a new line of research that will overcome a number of limitations inherent in early approaches. (This does not mean that those approaches should, or can, be ‘abolished’, just as Newtonian Mechanics was not set aside after Einstein, but rather, incorporated into the Einsteinian view.)
However, before offering new ideas, let us look into one old idea, and one long-running debate.
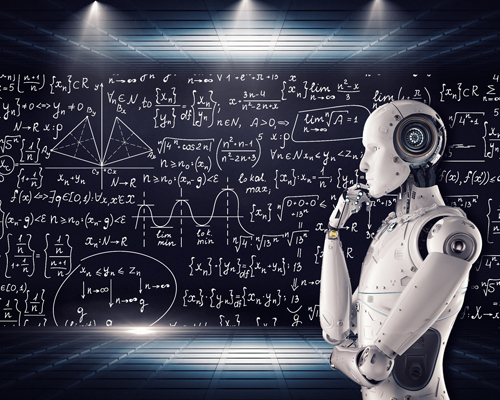
Artificial Intelligence Learning Mikemacmarketing 2018 Creative Commons 2
An Old Idea about Language
The old idea is the suggestion from Alan Turing that if a machine imitates intelligence so well that a large percentage of humans conversing with it by text alone can’t tell it is a machine, then it possesses intelligence. In fact, in ‘Computing Machinery and Intelligence’ (Mind, 1950), Turing identified several areas as representing the ‘highest manifestations’ of human intelligence. His examples included the study of languages (and translations); games (chess, etc.); and, mathematics and cryptography (including solving riddles). If in these areas the output of a computer cannot be distinguished from that of a human then its level of thinking is equivalent to that of a human, and so we can say that we’re dealing with an intelligent machine. According to Turing, the high-level, intellectual functions of the human brain can be reproduced in a computer without the computer precisely imitating the functioning of the brain.
It is noteworthy that only a couple of years after that publication, Walter Gray's ‘turtles’ appeared. These quite primitive robots showed surprisingly ‘intelligent’ behaviour. They could, for example, find their base station by orienting themselves towards light. This ability was born in direct interaction between the world and the simplest programming of the robots, and if Turing had written his paper after this debut, he would certainly have formulated the problem differently. However, it was his paper of 1950 that laid the foundations for the linguistic orientation of generations of artificial intelligence researchers. Turing himself admitted that comprehensive knowledge of the world is impossible without direct interaction with it. However, at that time, the idea of artificially imitating such activities as sports, eating, or sex, seemed unthinkable. Therefore, the British mathematician left those behaviours for an indefinitely distant future, suggesting to instead focus for now on games, languages and cryptography. As a result, Turing initiated a kind of human-machine race that has encouraged the development of systems performing narrow functions, be it a game of chess, translating, or driving a car better than a human being.
For his test, Turing was drawing on a Victorian ‘imitation game’. Here the judge must decide who of the players is a woman and who is pretending to be one, only by exchanging notes with the players. Obviously, the judge should not see the participants: they are separated from them by a wall or a screen. Turing transferred this situation to a computer trying to imitate a real person, also remaining hidden from the judge by a ‘wall’. The ‘wall’ deprives us of the physical embodiment of our conversation partner, and reduces ‘his’ responses to a limited set of verbal processes.
A Long-Running Debate about Language
In a prominent article, ‘Do Large Language Models Understand Us?’ (Medium, 2021), Blaise Aguera y Arcas considered if the successful teaching of deaf-blind-mute children is evidence that verbal communication could be the basis for developing artificial intelligence without needing embodied intelligence. This reminded us of a heated discussion dating back to the end of the Seventies, which one of the authors of this article, D. Dubrovsky, initiated, and directly participated in.
Besides the well-known scientific and technical achievements of the USSR, such as a manned space flight and nuclear energy, Soviet propaganda announced that the USSR had developed its own effective teaching technique for deaf-blind-mute kids, in the so-called ‘Zagorsk experiment’. Here teachers showed not only that the students could form social skills, but that they could have a fulfilled intellectual life. During the experiment, four students of Zagorsk Boarding School For The Deaf-Blind entered the Faculty of Psychology of Moscow State University and successfully graduated. Two of them even defended dissertations.
This could have been a truly significant pedagogical achievement if evidence of falsification hadn’t come out. All four participants were said to have been totally deaf-blind-mute from birth and completely devoid of not only language but conscious thought, and indeed any manifestations of psyche whatsoever. However, as it turned out, they had all lost their sight and hearing abilities fairly late in infancy, already possessing the full power of consciousness and speech. Moreover, two of them retained some hearing ability, and the other two retained some visual ability – enough to independently travel round the city on public transport!
Communist ideologists and a number of philosophers – creators of the technique among them – stated that the Zagorsk experiment proved that the Marxist concept of the formation of personality is correct. From that point of view, genetic factors play no role: everything is determined solely by social factors. Drawing on the Marxist maxim that ‘being determines consciousness’, it was assumed that a Marxist teacher could ‘sculpt’ the consciousness and personality of his student literally from scratch. Put simply, the Marxists used the Zagorsk experiment as a proof that it was possible to educate anyone from a ‘clean slate’ state – thus postulating a false dichotomy of nature versus nurture and in particular denying the role of biological and genetic factors in education. According to this Marxist approach, the most important thing for the intellectual development of a person is learning vocabulary and being able to communicate with other people using words.
These Marxist conclusions were sharply criticized by a number of philosophers, the central part in this specific debate being played by Dubrovsky. It was shown that biological, genetic factors play a fundamental role in the rehabilitation of the deaf-blind-mute. In the case of the loss of vision and hearing early in life, touch remains the main channel of communication for a child with the outside world, as well as some communication using smell and taste. However, the crucial role here concerns the genetic inclinations of children to the development of language, which also contribute to their overall sensitivity. A vivid example of this is provided by the upbringing and education of Helen Keller, who lost both her sight and hearing at the age of nineteen months, but, as is well-known, reached an exceptionally high level of intellectual development becoming a noted author, activist and lecturer.
It is noteworthy that even the deaf-blind-mute who have mastered spoken and written speech and reached a significant level of intellectual development, continue to rely on sign language and the sense of touch for communication and exploring the physical environment. They never stop practicing gestural communication. Therefore, using the supposed example of the deaf-blind-mute children as programmable ‘blank slates’ can hardly be definitive in AGI research, in which language is considered in more ‘disembodied’ terms.
It is also important to point out that knowing how to use a language does not in itself mean having intelligence in the true human sense of the word – of being able to consciously think about things. In AI, language is rather a tool for interacting with other things, and with people. By contrast, in explicitly conscious terms, language is a tool for expanding and deepening the understanding of one’s self, other people, of physical, biological, social phenomena, and of all kinds of causal and functional relations in the world around us. For conscious beings, language brings the ability to generalize, to abstract, analyze, and synthesize – that is, the ability to think. The agent ascribed with ‘true’ intelligence must possess all these qualities, as well as self-reflective ability. Moreover, real (conscious) intelligence is also based on the ‘dark matter’ of non-verbal perception and communication, and various subconscious processes. All this must be taken into account when we talk about language, intelligence, and creating AGI.
A Modern Discussion about Language & AI
In the aforementioned article by Aguera y Arcas, the issues of language, thinking, and having intelligence are considered from the perspective of developing deep learning through neural networks. Neural nets have paved the way for some outstanding results in the field of language processing and generation. Arcas opposes those researchers who believe that intelligence, in the sense of the capacity to understand the content of text or one's own actions, cannot be attributed to deep learning language models. They say that language models are just big statistical machines that map certain outputs (‘answers’) to certain inputs (‘questions’). Even though this obviously helps solve a number of practical problems quite successfully, this does not mean understanding either abstract or concrete concepts as a human would. But it is noteworthy that those criticizing Arcas for holding that language understanding is evidence of consciousness in machines, do not deny the achievements of deep learning language models for acquiring some sort of fundamental intelligence.
There are a few arguments put forward against large language models possessing the capacity to understand. For example, if an artificial intelligence is not embodied, has no physical presence, and cannot sense the world in a multimodal way as humans do, then its understanding of language must be insufficient, to say the least.
Arcas argues that our linguistic understanding is self-sufficient (‘complete’) because it is based on our innate and acquired knowledge as well as the rich sensory experience we have, and so opens up unlimited possibilities for learning. Through language we also have access to socially-determined perceptions (ie, to culture), richer in comparison to raw sensory experience that is not refined through language. Therefore, language itself is able to compensate for the weakness or lack of certain sensory abilities. It is in this context that Arcas refers to the experience of Helen Keller and the education of deaf-blind-mutes. However, Arcas’s arguments are short of the mark, since the success of Keller's education was based on the use and development of her available sensory abilities. This is precisely what the title of Helen Keller's famous essay illustrates: ‘I Am Blind – Yet I See; I Am Deaf – Yet I Hear’. Yet, although AI can detect the world through, for example, cameras or microphones, the idea that these computers actually experience sensations is much more difficult to justify. Generally speaking, it is hard to agree with Arcas’s statement that language can fill the sensory gap between humans and artificial intelligence, as well as with his interpretation of sequence learning in large language models, which is key to understanding conscious intelligence.
Arcas’s main points were critically reviewed in Melanie Mitchell's article, ‘What Does It Mean for AI to Understand?’ (Quanta Magazine, 2021). She writes, “The crux of the problem, in my view, is that understanding language requires understanding the world, and a machine exposed only to language cannot gain such an understanding.” Mitchell also notes that there are a lot of unexplained mechanisms involved in the processing of human speech, as linguistic research confirms. Artificial intelligence could not possibly understand language in the human sort of sense without this kind of ‘infrastructural’ background. Mitchell also says that, contrary to how Arcas interprets the argument concerning educating the deaf-blind, Hellen Keller's essay proves that both sensory experience and embodiment are paramount to consciously understanding language.
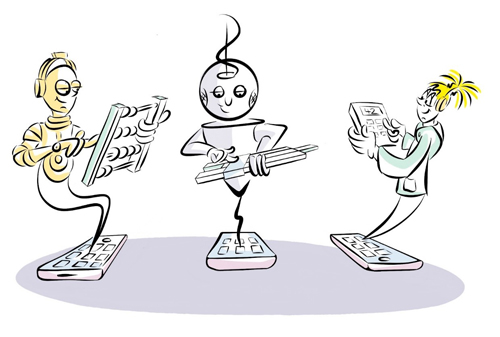
Artificial Agents Robert Wagner 2022 Creative Commons 4
Historic Requirements for Language & Sentience
What’s the connection between the modern discussions about artificial general intelligence and old debates about language and the nature of consciousness?
It turns out that transitioning deaf-blind-mute children from simple practical skills to intellectual communication using speech or the Braille alphabet always goes through gestural communication, and gestural communication always remains a part of communication for these people. Gestural or tactile communication is generally a proto-linguistic stage, a pre-verbal communication. For many deaf-blind students from the Zagorsk experiment it remained the main form of communication. This is important for artificial intelligence because it shows how intelligence is a complex biological product. This product is embodied intelligence, reliant on the ‘dark matter’ of non-verbal communication (such as body language). This indicates that a real thinking machine would have to be a product of a multi-dimensional interaction with people and with the outside world, both verbal and non-verbal, occurring both in a virtual and in a real environment. Yet the classic Turing Test, like the Winograd schemas and most other popular tests for artificial intelligence, cover only areas of verbal-virtual interaction. They all lie within the methodological paradigm set by Turing, and are still behind a ‘wall’ of virtuality. To break the wall would mean to enter the field of physical, sensation-filled exploration of the world by the growing artificial intelligence. After all, we understand that many animals have forms of consciousness, including cephalopods such as octopuses, for which thinking and its manifestations turn out to be connected with real living conditions – with the corporeality of living being. Furthermore (as has been emphasized by Dubrovsky), the mind arises in the course of biological evolution only in those organisms that actively move in the environment – that is, in animals, not plants. It seems then that comprehensive knowledge of the surrounding world is impossible without physically interacting with it. Therefore, one condition for creating a general artificial intelligence is the capacity to work in different modalities in different environments. This requires access to the non-verbal and the physical.
Examples of artificially intelligent agents that cope with non-verbal tasks are systems that can play computer games; or the virtual TV presenter Elena, created at the Sber Robotics Laboratory. Elena is capably of imitating a real TV presenter, including movements, facial expressions, emotional expressions and other gestures. However, neither of these examples leave the limits of the virtual. Real interaction with the physical world is still an extremely difficult task to build into artificial intelligence. In the case of AGI, this kind of machine must comprehend all four areas of interaction (movements, facial expressions, emotional expressions, and gestures), as well as working with environments.
The Advent of Techno-Umwelts
Back in the nineteenth century, the biologist Jakob von Uexküll pointed out that different living beings have different spheres of world perception – different umwelts. The umwelt of a butterfly is very different from that of a fish, or from that of a person, for example. The umwelt of a person is of course well-known to each of us.
By analogy, we propose to call four areas of interaction possible for machines ‘techno-umwelts’. A ‘techno-umwelt’ would be the domain of perception for a machine: how a machine perceives the world. Many of us have seen visualizations of the techno-umwelts of unmanned vehicles using radars and lidars in videos, for example. But the two dimensions of interactions described above – verbal/non-verbal, and virtual/physical – give four possible techno-umwelts, or areas of perception for a machine: 1) Verbal virtual; 2) Non-verbal virtual; 3) Verbal physical; and 4) Non-verbal physical. The versatility that marks general or comprehensive intelligence, that is, AGI, would only be possible when the machine freely operates in all four of these techno-umwelts.
Current AI systems are capable of coming to recognize objects of different classes without having been programmed to do so. This is a major achievement, but it has nothing to do with generality, which we will now define as the capability of an agent to work in different umwelts. So in order to achieve generality for an intelligent agent, it will be necessary to implement ‘translators’ between the language of one domain of world perception and the language of another. Only then could artificial intelligence become truly multimodal – meaning, it will be able to solve a wide range of possible tasks and comprehensively communicate with a human.
The idea of the combination of techno-umwelts thus gives us the opportunity to propose a new definition of AGI:
Artificial general intelligence is the ability of a robot (a machine with sense-think-act capability) to learn and act jointly with a person or autonomously in any techno-umwelt (but potentially better than a specialist in this field), achieving the goals set in all four techno-umwelts, while limiting the resources consumed by the robot.
As this multidimensional ability emerges it will forever change the way we interact with technology. After millennia of philosophical reflection, and centuries of scientific and technological progress, for the first time in history, people will encounter truly smart non-human things - devices that may come to have even more complete and accurate knowledge about the world and about us than human being themselves. This situation will call for a new outlook on what a person and a mind are, as well as a redefinition of many other established ideas. The redefinition has already begun.
On the one hand, we are beginning to ‘dissolve’ into the technologies and virtual worlds surrounding us, blurring the concept of ‘human’. On the other hand, as computers explore new areas of activity, be it chess or machine translation or whatever else, those areas are no longer exclusive to humans. Perhaps humans are the final frontier that the machine cannot yet overcome.
© A. Efimov, D. Dubrovsky and F. Matveev 2023
A. Efimov is Chair of Engineering Cybernetics at the National Science and Technology University MISIS, D. Dubrovsky is Chief Scientist at the Institute of Philosophy, Russian Academy of Science and F. Matveev is a student at San Francisco State University.