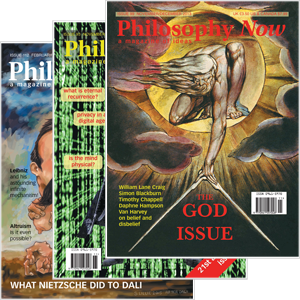
Your complimentary articles
You’ve read one of your four complimentary articles for this month.
You can read four articles free per month. To have complete access to the thousands of philosophy articles on this site, please
Machine Morality
How Machines Can Advance Ethics
Susan Leigh Anderson and Michael Anderson relate how their attempts to build ethical machines have advanced their understanding of ethics.
Our current research is concerned with the newly emerging field of machine ethics. Unlike computer ethics, which has traditionally focused on ethical issues surrounding humans’ use of machines, machine ethics is concerned with ensuring that the behavior of the machines themselves is ethically acceptable. This requires that ethical decision-making be computable, at least to some degree.
Attempting to make ethical decision-making computable requires, first, that we settle on an existing ethical theory, or at least an approach to ethical decision-making that appears to have merit. After that, the theory or approach must be made precise enough to be programmed into a machine. This involves cooperation between ethicists and AI researchers. We also believe that attempting to implement computerised ethical decision-making could lead to advances in ethics, perhaps even leading to the development of new theories, as AI researchers force scrutiny of the details involved in actually applying a theory or approach to particular cases where machines must make ethical decisions. As Daniel Dennett stated in a talk at the International Computers and Philosophy Conference, Laval, France in 2006: “AI makes Philosophy honest.”
Since we are concerned with the behavior of machines – their actions rather than their metaphysical status – we have adopted an action-based approach to ethical theory. No single-principle action-based theory is generally accepted. Teleological (or consequentialist) theories like Act Utilitarianism, which focus entirely on the likely consequences of actions, can justify the violation of human beings’ rights, by sacrificing one person for the greater net good. They can also conflict with our notion of justice – what people deserve – which depends on past behavior, not future consequences of actions. Deontological theories, such as Kant’s Categorical Imperative, where the rightness and wrongness of actions depends on precepts and rules rather than consequences, can emphasize the importance of rights and justice, but such theories can be accused of ignoring consequences.
Along with W. D. Ross in The Right and the Good (1930), we maintain that the best approach to ethical decision-making is one which combines elements of both teleological and deontological theories: the prima facie duty approach. Ross believed that there isn’t a single absolute duty to which we must adhere, but rather a number of duties we should try to follow – but each could be overridden in certain situations. We have aprima facie [‘first appearance’] duty, for instance, to follow through with a promise we’ve made; but if it causes great harm to do so, it may be overridden by another prima facie duty not to cause harm.
There are two advantages to this approach. First, it can be tailored to the domain one is concerned with. There may be different sets of prima facie duties for biomedical ethics, business ethics, journalistic ethics, legal ethics and the ethics of sports, for instance. Second, duties can be added or removed if it becomes clear that they’re needed or are redundant. The major drawback to this approach is that it needs to be supplemented with a principle for making decisions in cases when prima facie duties give conflicting advice. Consider an example: You promised your elderly parents that you would help them by cleaning out the overflowing gutters on their house today. Just as you are about to leave, a friend calls to say that her car has broken down some distance from your apartment and she needs a ride. She reminds you that you owe her a favor. What should you do? Determining the correct action involves correctly balancing prima facie duties of fidelity (to keep your promise to your parents), beneficence (to help your parents or your friend) and gratitude or reciprocity (to your friend, to whom you owe a favor).
Ross had no solution to this problem beyond using our intuition to decide which duty should prevail. That wouldn’t be helpful for a machine attempting to adopt this approach, and doesn’t seem satisfactory for human beings either. People may not have an intuition, or may have different intuitions, about which duty should be paramount in a particular situation, and they are likely to emphasize the duty which permits them to rationalize doing what serves their self-interest. We have worked on the problem of coming up with an objective principle or principles for determining the correct action when following the prima facie duty approach. The possibility that computers could help in solving this ‘decision principle problem’ – a solution to which is needed for a prima facie duty theory to ever be consistent and complete – was a major reason the ethicist of our team became interested in machine ethics.
Our solution to the decision principle problem was inspired by John Rawls’ ‘reflective equilibrium’ approach to creating and refining ethical principles (found in ‘Outline for a Decision Procedure for Ethics’, Philosophical Review vol. 60, 1951). His approach involves generalizing from intuitions about particular cases, testing those generalizations on further cases, and then repeating this process towards the end of developing a principle that agrees with intuition. In ‘An Approach to Computing Ethics’ (IEEE Intelligent Systems, Vol. 21, No. 4, 2006), we detailed how we used machine learning techniques to abstract a decision principle from specific cases of a common type of ethical dilemma involving a number of prima facie duties, where experts in ethics have clear intuitions about the correct action.
Starting with a test case, we used a well-known prima facie duty theory intended to cover dilemmas in the area of biomedicine, found in Beauchamp and Childress’ Principles of Biomedical Ethics (1979). Our considerations involved three duties: respect for the autonomy of the patient (ie the patient sufficiently understands his/her condition, and decisions are made free of external and internal constraints), nonmaleficence (not causing harm to the patient) and beneficence (promoting patient welfare). Further, the type of ethical dilemma we considered was one many healthcare professionals have faced: A doctor has recommended a particular treatment for her competent adult patient and the patient has rejected that treatment. Should she try to change the patient’s mind, or accept the patient’s decision as final? The dilemma arises because, on the one hand, the doctor shouldn’t challenge the patient’s autonomy unnecessarily, while on the other hand, she might have concerns about why the patient is refusing treatment – that is, whether the decision is fully autonomous. This dilemma also involves the duty not to cause harm to the patient (nonmaleficence) and/or to promote patient welfare (beneficence), since the recommended treatment is designed to prevent harm to and/or benefit the patient.
A Decision Principle For Conflicting Duties
In this dilemma, the doctor has just two options – either to accept the patient’s decision or not – and there are a finite number of specific types of such cases. The representation scheme we developed for these possible cases consists of a set of values for each of the possible actions, where these values reflect whether particular prima facie duties are satisfied or violated, and if so, to what degree. The reason we needed degrees is because there is an ethically-relevant difference between a strong affirmation or violation of, first, patient autonomy (fully supporting the patient’s decision to do what he wants, or forcing the patient to do what he does not want to do) and a weaker affirmation or violation (supporting or questioning a less than fully autonomous decision). Similarly, there is an ethically-relevant difference between a strong affirmation/violation of nonmaleficence (not allowing great harm to come to the patient) and a weaker one (not allowing some harm to come to the patient). Finally, we need to distinguish between a strong affirmation/violation of the duty of beneficence (allowing the patient to be greatly benefitted/allowing the patient to lose much benefit) versus a weaker one (allowing the patient to receive some benefit/permitting the patient to lose some benefit). We used -2 to represent a strong violation of a duty, -1 to represent a weaker violation, 0 when the duty is not involved, +1 for some affirmation and +2 for a strong affirmation of the duty.
Consider the following example of an ethical dilemma of the type described and how it’s represented numerically: Because of long-standing religious beliefs that don’t permit him to take medications, a patient refuses to take an antibiotic that is likely to prevent complications from his illness – complications that are not likely to be severe. The patient understands the consequences of this refusal. Should the doctor accept his decision, or try to convince him to take the antibiotic? In this case, accepting the patient’s decision involves +2 for respect for the autonomy of the patient, since it’s a fully autonomous decision, a -1 for nonmaleficence, since it will lead to some harm for the patient that could have been prevented, and -1 for beneficence, since the patient will lose some benefit that he could have received from taking the antibiotic. Questioning the patient’s decision, on the other hand, would involve a -1 for respecting patient autonomy, a +1 for nonmaleficence and a +1 for beneficence, since taking the antibiotic would lead to the patient avoiding some harm as well as benefitting him somewhat. From this we generate a case profile: Accept: +2, -1, -1; Try Again: -1, +1, +1.
In this and other dilemmas of this type, we took the ‘correct’ answers from a discussion of similar cases by Buchanan and Brock in their article ‘Deciding for Others’ in The Ethics of Surrogate Decision Making (1989). We believe there’s a consensus among bioethicists that these are the correct answers. For instance, in the present case, medical ethicists would say that one should accept the patient’s decision.
It turns out that with our range of values for the three possible duties at stake, there are 18 possible case profiles, where each profile represents a different ethical dilemma of the type considered. Furthermore, giving the computer the correct answer to just 4 of these profiles enables it, via machine learning techniques, to abstract a principle that gives the correct answer for the remaining 14 cases. The principle learned was the following: A doctor should challenge a patient’s decision if it isn’t fully autonomous and there’s either any violation of nonmaleficence or a severe violation of beneficence. We believe that this principle, although clearly implicit in the consensus judgments of the ethicists, has never before been explicitly stated. The principle is also supported by an insight of Ross’ that it is worse to cause harm to, or allow harm to come to someone, than not to help someone. We offer this as evidence that defining ethics more precisely will permit machine learning techniques to discover novel and useful principles in ethics.
We were able to use this principle derived by a machine in two applications we created. MedEthEx (Medical Ethics Expert), a medical ethical advisor system, uses this principle to give ethical advice to a user faced with a dilemma of the type we’ve described (see ‘MedEthEx: A Prototype Medical Ethics Advisor’ in The Proceedings of the Eighteenth Conference on Innovative Applications of Artificial Intelligence, 2006). EthEl (Ethical Eldercare System) determines when a patient should be reminded to take medication and when a refusal to do so is serious enough to contact a supervisor (notifying the supervisor corresponds to ‘trying again’ in the earlier dilemma). (See ‘Machine Ethics: Creating an Ethical Intelligent Agent’ in Artificial Intelligence Magazine, Vol. 28, 2007.)
Future Research
Besides only considering certain types of ethical dilemmas, in our work to date we have assumed a particular set of prima facie duties, and a narrow range of satisfaction/violation levels. In our future work we plan to lift those assumptions, and start with a minimal commitment: dilemmas involving prima facie duties will initially involve the satisfaction or violation of at least one duty. A commitment to at least one duty can be viewed as simply a commitment to ethics: there is a least one obligation incumbent upon the agent in any ethical dilemma. If it turns out that there’s only one duty, then there’s a single, absolute ethical duty the agent ought to follow. If it turns out that there are two or more potentially competing duties (as we suspect, and have previously assumed), then it will have been established that there are a number of prima facie duties that must be weighed in ethical dilemmas, giving rise to the need for a deciding ethical principle to resolve the conflicts. Furthermore, a range of duty satisfaction/violation levels is likely to be introduced, even increased, depending upon how many gradations are needed to distinguish between cases. We envision a type of machine learning process where the machine interacts with ethicists to generate features of ethical dilemmas, leading to prima facie duties, levels of their satisfaction/violation, as well as decision principles. New features, extra duties or a wider range of satisfaction/violation levels will be needed whenever the machine is given two ethical dilemmas appearing to have the same profile, but where different actions are recommended by ethicists. When this happens there must either be an ethically relevant feature in one of the dilemmas which is not in the other and which has not yet been revealed, or else a wider range of satisfaction/violation levels is needed.
One may have a concern about our approach: we are assuming that ethicists are in agreement about the right answer to at least some ethical dilemmas. Our approach depends upon there being specific cases in which there is such agreement, and we’re trying to use machines to abstract principles that reflect ethicists’ views about those cases.
What if no such agreement exists? Three responses can be given to this concern, two from the ethicist’s perspective and one from AI’s perspective:
(1) Most ethicists reject Ethical Relativism – the view that there aren’t single correct answers to ethical dilemmas – primarily because this view entails that one cannot criticize the actions of individuals when they act in accordance with their own beliefs, or societies whose actions are approved by the majority, no matter how heinous they are. Against the response that Ethical Relativism is more tolerant than its converse, Ethical Absolutism, it has been pointed out that Ethical Relativists cannot say that anything is absolutely good – even tolerance. To justify their stance, Relativists tend to focus on contentious issues that have yet to be resolved (eg abortion), not appreciating enough the consensus that has emerged for many other issues. For instance, it is now generally accepted where it once wasn’t that slavery is morally wrong, and that one’s race or sex does not justify being treated differently.
Admittedly, we began testing our approach using a type of medical ethical dilemma partly because there is more consensus in biomedical ethics than in other areas. Biomedical ethics arose out of a need to resolve pressing problems faced by doctors, nurses, insurers, hospital ethics boards and medical researchers. As a result there has been much discussion about specific cases, which has led to a consensus emerging as to what is best in these cases. But generally, ethicists see completeness in an ethical theory (its ability to determine the correct answer to all ethical dilemmas) as a goal for which to strive rather than something to expect now. The ethical theory or framework for resolving ethical disputes that we adopt should therefore allow for updates, as issues that once were considered contentious are resolved.
(2) It is very possible that a framework for handling ethical dilemmas that reveals the features of those dilemmas and the intensities of those features will lead to a resolution to dilemmas now considered contentious. If not, it will at least reveal the precise nature of the disagreement among ethicists. In either case, such work will lead to advances in ethical theory.
(3) From the AI perspective, we should probably not allow machines to engage in actions where there is not a consensus among ethicists as to the correct way to behave. Machines’ contact with humans should be limited to activities where moral consensus exists, even if it means that we cannot use them as some would like. We can know where the boundaries are simply by seeing where there is consensus and where there is not.
We hope that this brief introduction to our research within the new, interdisciplinary field of machine ethics has shown that machine ethics has the potential to lead to advances in applied and theoretical ethics. Work revealing the extent to which ethical decision-making can made precise enough to be computable will help clarify the nature of one of the most important fields of philosophy.
© Dr Susan Leigh Anderson and Dr Michael Anderson 2009
Susan Leigh Anderson is in the Philosophy Department at the University of Connecticut. Michael Anderson is in the Computer Science Department at the University of Hartford.