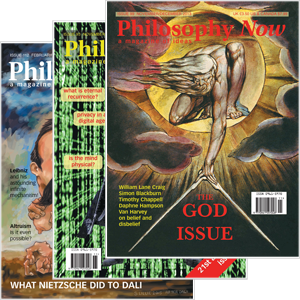
Your complimentary articles
You’ve read one of your four complimentary articles for this month.
You can read four articles free per month. To have complete access to the thousands of philosophy articles on this site, please
Books
The Promise of Artificial Intelligence by Brian Cantwell Smith
Joshua Schrier asks whether artificial real intelligence is possible.
Can thinking be reduced to a series of logical rules which can be performed by anyone, even a machine? Enlightenment philosophers, including Gottfried Leibniz, Thomas Hobbes, and René Descartes, all considered the possibility of mechanical approaches to thinking. In our own era, computer technology has enabled artificial intelligence algorithms to outperform humans in tasks as diverse as playing chess, flying airplanes, diagnosing diseases, and recognizing cat pictures on the internet, among many others. Some have speculated that just a little bit more computer power would enable these algorithms to achieve general human-level, or perhaps even super-human, intelligence. Or is there instead a fundamental roadblock to human-level artificial intelligence?
In this engaging and conversational book, Brian Cantwell Smith, who is Reid Hoffman Professor of Artificial Intelligence and the Human at the University of Toronto, argues that no current or proposed forms of AI lead to genuine intelligence. According to Smith, the barrier is not a lack of computing power, but rather the ontological and epistemic realities about how electronic algorithms engage with and in the world, as I’ll explain.
Smith distinguishes between ‘reckoning’ and ‘judgement’ as forms of thinking. Reckoning – the outcome of a sequence of calculations – can be quite sophisticated, and in many cases computers already exceed human capabilities in this regard; for example, certain chess programs can beat any human player. In contrast, judgement refers to dispassionate and deliberate thought, guided by ethical commitments, resulting in actions appropriate to the situation. In Smith’s view, much of what we call ‘intelligence’ is really using judgement in this sense to operate skillfully in uncertain, underdefined environments, in an appropriate way. It’s more like the ancient Greek concept of phronesis or practical virtue, than a deductive logical process. Smith argues that all existing and planned AI approaches are limited to reckoning and not capable of judgement.
He begins his analysis with an abbreviated review of various AI approaches, their philosophical assumptions, and the limitations imposed by those assumptions. ‘First wave’ AI (also termed ‘good old-fashioned AI’ by the philosopher John Haugeland) assumed that thought consists of performing symbolic operations on well-defined pre-existing object-symbols. (It was an electronic version of the ‘reasoning as calculation’ model put forth by Thomas Hobbes in De Corpore in 1655.) By the late 1970s it was clear that this approach had reached its limits. Aside from the practical difficulty of recognizing distinct objects from the raw sensor data (the information input to the AI through cameras, for instance), there are many ways in which the world doesn’t in any case either actually (ontologically) or computationally meaningfully (epistemically) consist of discrete, neatly separated objects. So, although this first wave of AI could prove geometry theorems or play a passable game of chess, it fell short in everyday organic tasks like recognizing cat pictures. Second wave AI (a.k.a. ‘machine learning’) addresses the immediate practical difficulties of reckoning the nature of the environment from the sensor data, including working out the locations and shapes of objects, but not the deeper judgement-related issues stemming from participating as another object in the world. Machine learning approaches ‘learn’ the patterns needed to classify objects, or at least, learn digital values associated with objects from a mass of raw sensor data. Think of a computer learning to recognize cats from pixels in photographs. In contrast to first-wave AI, machine learning uses statistical inferences conducted in just a few logical steps, but these are built up using large numbers of examples, involving a large number of weakly correlated variables. For example, using millions of cat photos, the individual pixels of which are not in themselves obviously related to ‘cat’, the system will teach itself to recognise photos with cats, compared with ones without cats. However, despite the impressive performance of these algorithms, they still fall into Smith’s ‘reckoning’ category.
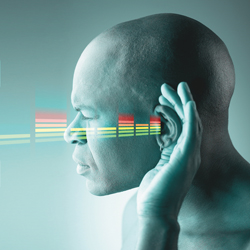
The problem preventing computers from developing judgement, according to Smith (who in turn is quoting Haugeland), is that even second wave AI algorithms don’t ‘give a damn’ – that is, they do not have ‘skin in the game’ of the results of reckoning outcomes. The outcome of the reckoning means nothing to them – nor is it clear what would be necessary for it to do so. No existing algorithms would or could even in principle balk at the impossibility of a given input, or express moral outrage at the outcome. It is one thing to reckon that a collection of pixels should be labeled as a ‘cat’, but quite another to refuse to make that classification because it would then become the main course on the dinner menu, and thus violate a personal or social norm. It is one step even further to make this refusal while knowing and caring that it might upset one’s host (creating a social harm) or make them go hungry (creating a personal bodily harm). All these conclusions require contextual judgements and a calculus of consequences appropriate to embodied agents. These requirements are lacking in any proposed AI scheme.
From an ethical – and also from a legal – perspective, having some sort of stake in an outcome is necessary for being accountable for a decision about the outcome. Smith says that making this sort of decision is essential to judgement as a form of genuine intelligence, too. He argues that merely adding more data or more computing power to the current approaches cannot change this fundamental lack that flows through the current AI research agenda. It cannot change mere reckoning into judgement.
For Smith, all living creatures, regardless of the sophistication of their reckonings about the world, have some commitment to the world. Indeed, their decisions may have literal life-or-death consequences. In contrast to their current artificial counterparts, this ‘will to live’ (pace Arthur Schopenhauer) holds living organisms genuinely accountable to object registrations as the basis of their judgement. In other words, if an organism’s reaction to the world is not based upon a realistic picture of what’s happening in it, it’s in real trouble! Thus, the problem with putting judgement in a machine may not be that AI lacks sufficient brains to make a decision, but rather that it lacks a body to suffer the consequences.
This is a particularly interesting argument for philosophical readers interested in ontology, epistemology, consciousness or ethics (particularly virtue ethics). Smith is explicitly sympathetic to materialist theories of mind, and states on several occasions that his arguments do not theoretically exclude the existence of genuinely intelligent AI. Being ‘artificial’ is not the problem, he argues; the problem is rather that current approaches to AI lack the necessary commitment to creating an artificial comprehension of the reality of objects, and creating a real, involved, deciding agent in a shared world.
© Joshua Schrier 2021
Joshua Schrier is Kim B. and Stephen E. Bepler Chair Professor of Chemistry at Fordham University in New York.
• The Promise of Artificial Intelligence: Reckoning and Judgment, Brian Cantwell Smith, 2019, MIT Press, 184 pages, £13 pb, ISBN 9780262043045